Normalizing the Use of Single-Item Measures: Validation of the Single-Item Compendium for Organizational Psychology
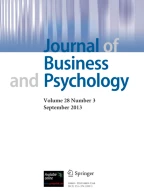
The application of single-item measures has the potential to help applied researchers address conceptual, methodological, and empirical challenges. Based on a large-scale evidence-based approach, we empirically examined the degree to which various constructs in the organizational sciences can be reliably and validly assessed with a single item. In study 1, across 91 selected constructs, 71.4% of the single-item measures demonstrated strong if not very strong definitional correspondence (as a measure of content validity). In study 2, based on a heterogeneous sample of working adults, we demonstrate that the majority of single-item measures examined demonstrated little to no comprehension or usability concerns. Study 3 provides evidence for the reliability of the proposed single-item measures based on test–retest reliabilities across the three temporal conditions (1 day, 2 weeks, 1 month). In study 4, we examined issues of construct and criterion validity using a multi-trait, multi-method approach. Collectively, 75 of the 91 focal measures demonstrated very good or extensive validity, evidencing moderate to high content validity, no usability concerns, moderate to high test–retest reliability, and extensive criterion validity. Finally, in study 5, we empirically examined the argument that only conceptually narrow constructs can be reliably and validly assessed with single-item measures. Results suggest that there is no relationship between subject matter expert evaluations of construct breadth and reliability and validity evidence collected across the first four studies. Beyond providing an off-the-shelf compendium of validated single-item measures, we abstract our validation steps providing a roadmap to replicate and build upon. Limitations and future directions are discussed.
This is a preview of subscription content, log in via an institution to check access.
Access this article
Subscribe and save
Springer+ Basic
€32.70 /Month
- Get 10 units per month
- Download Article/Chapter or eBook
- 1 Unit = 1 Article or 1 Chapter
- Cancel anytime
Buy Now
Price includes VAT (France)
Instant access to the full article PDF.
Rent this article via DeepDyve
Similar content being viewed by others
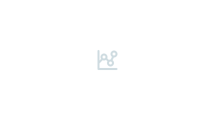
Reporting reliability, convergent and discriminant validity with structural equation modeling: A review and best-practice recommendations
Article Open access 30 January 2023
Four Research Designs and a Comprehensive Analysis Strategy for Investigating Common Method Variance with Self-Report Measures Using Latent Variables
Article 17 December 2015
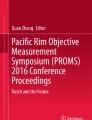
Research Design Considerations in Human Science Research: Reconciling Conceptions of Science, Theories of Measurement and Research Methods
Chapter © 2018
Explore related subjects
Change history
Notes
We should note that definitional and terminological confusion surrounds the concepts of complexity and concreteness, with different authors approaching and defining terms in inconsistent ways. For example, referring to a construct as “broad” or “abstract” could be meant to refer to how large the construct space is (e.g., a “complex” personality trait), to denote that a construct is multi-dimensional in nature (e.g., job satisfaction), or to denote that a concept is not grounded in sensory-motor information (e.g., role ambiguity; Borghi et al. 2017).
We recognize that content validity is also often conceptualized in terms of the degree to which a construct is accurately captured by the item(s) included in a given measure. While we follow the example set forth by Colquitt et al. (2019) in terms of examining definitional correspondence as an indicator of content validity, in other literatures, our approach might be evaluated as an examination of face validity. As discussed by Allen et al., (2022, p. 1) though, “just as for multi-item measures, it is critically important for single-item measures to demonstrate face validity,” wherein face validity can be defined as the “clarity or relevance” of an item or measure.
Colquitt et al. (2019) provide overall criteria with five levels (Table 5 in their study). A definitional correspondence estimate of .91 and above is considered very strong, .87 to .90 is strong, .84 to .86 is moderate, .60 to .83 is weak, and .59 and below as lack of definitional correspondence. To be clear, we are not arguing that a definitional correspondence estimate of .60 to .83, which Colquitt et al. again define as weak, is necessarily acceptable. Consistent with our overarching argument for triangulating the validity of single-item measures, the goal is to ensure content validity is demonstrated first and foremost and then evaluated against other pieces of psychometric evidence relative to the needs of a specific program of research.
Please note that presenting multiple examples in an item does not mean an item is necessarily double-barreled. Double-barreled questions ask about two distinct (i.e., divergent) “attitudinal” phenomena wherein respondents provide only one answer (Olson 2008). Using the word “and” in an item does not inherently make it a double-barreled item. However, in the spirit of study 2, the use of multiple examples (conjuncts) may increase the complexity and impact the interpretability of single-item measures (Olson 2008).
Cicchetti (1994) suggests that ICC values greater than .74 indicate excellent reliability, between .60 and .74 indicate good reliability, between .40 and .59 indicate fair reliability, and below .40 indicate poor reliability.
To be clear, similar to Hypothesis 1, we are not advocating that single-item measures demonstrating “poor” construct validity (i.e., loadings of .32 to .44) are necessarily valid. We set this minimum based on accepted practices in the larger scale development literature. As noted by Allen et al. (2022) though, standards applied to validating single-item measures may be different than those used for multi-item measures. Thus, depending on the construct under consideration, setting more stringent minimums might be prudent. It should also be recognized that using different multi-item measures of the same focal construct might result in different construct validity estimates for a given single-item measures. Again then, single-item construct validity evidence must be interpreted relative to other pieces of validity evidence including content validity as well as the psychometric characteristics of the comparative multi-item measure.
These two multi-item measures included negatively worded items. Autonomy climate (χ 2 (5) = 83.04, p < .001, CFI = .83, SRMR = .07) and perceived contract breach (χ 2 (5) = 110.48, p < .001, CFI = .89, SRMR = .08) both continued to demonstrate poor fit when the respective single-item measure was excluded and the CFA model re-estimated; the poor fit seems to be a function of the multi-item measure, not because of single-item measure.
Another way to evaluate construct validity is to examine the bivariate correlation between the two measurement approaches. As such, the bivariate correlations between the single-item and multi-item reference measure, for each construct, are reported in Table 2. The average construct validity correlation across the 91 constructs was .58 (SD = .19). Interestingly, across the 91 constructs, single-item CFA factor loadings correlated at .97 (p < .001) with the observed bivariate correlations between the single-item and multi-item construct measures suggesting that the two approaches for establishing construct validity are effectively equivalent.
Relatedly, in an effort to reduce the trade-off between the number of items in the scale length and the scale quality, Cortina et al. (2020) have developed a procedure that aims to optimize the scale quality (e.g., alpha reliability coefficient, part-whole correlations) of the resulting shortened scale by analyzing all possible sets of items drawn from the full scale.
We established construct validity evaluations based on a point system; we assigned points based on content validity (i.e., 1 point: content validity ≤ .69, 5 points: content validity ≥ .90), amount of usability concerns (i.e., 1 point: systematic usability concerns, 4 points: no meaningful usability concerns), average ICC test–retest reliability scores (i.e., 1 point: ICC < .40, 4 points: ICC >.74), construct validity (i.e., 0 points: CFA factor loading < .32, 5 points: CFA factor loadings >.70), and criterion validity (i.e., 1 point: limited to no evidence of criterion validity, 5 points: systematic evidence of criterion validity). We then computed an average across these different pieces of reliability and validity (scores ranged from 1.67 to 4.47). Constructs with scores greater than 4.00 were evaluated as having extensive construct validity, constructs between 3.00 and 3.99 were evaluated as demonstrating very good construct validity, constructs between 2.70 and 2.99 were evaluated as demonstrating good construct validity, constructs between 2.25 and 2.69 were evaluated as demonstrating limited construct validity, and constructs less than 2.25 were evaluated as demonstrating no construct validity. Additional information is available upon request.
There was also no relationship between SME ratings of construct breadth and the final triangulated construct validity level reported in Table 3 (r = 0.15, p = 0.14).
References
- Aguinis, H., Hill, N. S., & Bailey, J. R. (2021). Best practices in data collection and preparation: Recommendations for reviewers, editors, and authors. Organizational Research Methods,24, 678–693. ArticleGoogle Scholar
- Allen, M. S., Iliescu, D., & Greiff, S. (2022). Single item measures in psychological science. European Journal of Psychological Assessment,38(1), 1–5. ArticleGoogle Scholar
- Anderson, J. C., & Gerbing, D. W. (1991). Predicting the performance of measures in a confirmatory factor analysis with a pretest assessment of their substantive validities. Journal of Applied Psychology,76(5), 732–740. ArticleGoogle Scholar
- Ang, L., & Eisend, M. (2018). Single versus multiple measurement of attitudes: A meta-analysis of advertising studies validates the single-item measure approach. Journal of Advertising Research,58(2), 218–227. ArticleGoogle Scholar
- Arnulf, J. K., Larsen, K. R., Martinsen, Ø. L., & Egeland, T. (2018). The failing measurement of attitudes: How semantic determinants of individual survey responses come to replace measures of attitude strength. Behavior Research Methods,50(6), 2345–2365. ArticlePubMedGoogle Scholar
- Bergkvist, L., & Rossiter, J. R. (2007). The predictive validity of multiple-item versus single-item measures of the same constructs. Journal of Marketing Research,44(2), 175–184. ArticleGoogle Scholar
- Borghi, A. M., Binkofski, F., Castelfranchi, C., Cimatti, F., Scorolli, C., & Tummolini, L. (2017). The challenge of abstract concepts. Psychological Bulletin,143(3), 263–292. ArticlePubMedGoogle Scholar
- Bowling, N. A., Gibson, A. M., Houpt, J. W., & Brower, C. K. (2021). Will the questions ever end? Person-level increases in careless responding during questionnaire completion. Organizational Research Methods,24, 718–738. ArticleGoogle Scholar
- Boyd, B. K., Gove, S., & Hitt, M. A. (2005). Construct measurement in strategic management research: Illusion or reality? Strategic Management Journal,26(3), 239–257. ArticleGoogle Scholar
- Boyle, G. J. (1991). Does item homogeneity indicate internal consistency or item redundancy in psychometric scales? Personality and Individual Differences,12(3), 291–294. ArticleGoogle Scholar
- Callegaro, M., Manfreda, K. L., & Vehovar, V. (2015). Web survey methodology. Sage. BookGoogle Scholar
- Campbell, D. T., & Fiske, D. W. (1959). Convergent and discriminant validation by the multitrait-multimethod matrix. Psychological Bulletin,56, 81–105. ArticlePubMedGoogle Scholar
- Casper, W. J., Vaziri, H., Wayne, J. H., DeHauw, S., & Greenhaus, J. (2018). The jingle-jangle of work–nonwork balance: A comprehensive and meta-analytic review of its meaning and measurement. Journal of Applied Psychology,103(2), 182–214. ArticlePubMedGoogle Scholar
- Čehovin, G., Bosnjak, M., & Lozar Manfreda, K. (2018). Meta-analyses in survey methodology: A systematic review. Public Opinion Quarterly,82(4), 641–660. ArticleGoogle Scholar
- Cheah, J. H., Sarstedt, M., Ringle, C. M., Ramayah, T., & Ting, H. (2018). Convergent validity assessment of formatively measured constructs in PLS-SEM. International Journal of Contemporary Hospitality Management,30(11), 3192–3210. ArticleGoogle Scholar
- Cicchetti, D. V. (1994). Guidelines, criteria, and rules of thumb for evaluating normed and standardized assessment instruments in psychology. Psychological Assessment,6(4), 284–290. ArticleGoogle Scholar
- Colquitt, J. A., Sabey, T. B., Rodell, J. B., & Hill, E. T. (2019). Content validation guidelines: Evaluation criteria for definitional correspondence and definitional distinctiveness. Journal of Applied Psychology,104(10), 1243–1265. ArticlePubMedGoogle Scholar
- Comrey, A. L., & Lee, H. B. (1992). A first course in factor analysis (2nd ed.). Lawrence Erlbaum Associates, Inc.
- Cortina, J. M., Sheng, Z., Keener, S. K., Keeler, K. R., Grubb, L. K., Schmitt, N., & Banks, G. C. (2020). From alpha to omega and beyond! A look at the past, present, and (possible) future of psychometric soundness in the Journal of Applied Psychology. Journal of Applied Psychology,105(12), 1351–1381. ArticlePubMedGoogle Scholar
- Cronbach, L. J., & Meehl, P. E. (1955). Construct validity in psychological tests. Psychological Bulletin,52(4), 281–302. ArticlePubMedGoogle Scholar
- Crossley, S. A., Greenfield, J., & McNamara, D. S. (2008). Assessing text readability using cognitively based indices. Teachers of English to Speakers of Other Languages Quarterly,42(3), 475–493. Google Scholar
- Curran, P. G. (2016). Methods for the detection of carelessly invalid responses in survey data. Journal of Experimental Social Psychology,66, 4–19. ArticleGoogle Scholar
- De Vries, R. E., Realo, A., & Allik, J. (2016). Using personality item characteristics to predict single-item internal reliability, retest reliability, and self-other agreement. European Journal of Personality,30(6), 618–636. ArticleGoogle Scholar
- Dormann, C., & Van de Ven, B. (2014). Timing in methods for studying psychosocial factors at work. In M. Dollard, A. Shimazu, R. B. Nordin, P. Brough, & M. Tuckey (Eds.), Psychosocial factors at work in the Asia Pacific (pp. 89–116). Springer.
- Drolet, A. L., & Morrison, D. G. (2001). Do we really need multiple-item measures in service research? Journal of Service Research,3(3), 196–204. ArticleGoogle Scholar
- DuBay, W. H. (2004). The principles of readability. Impact Information, Costa Mesa, CA.
- Edwards, J. R. (2003). Construct validation in organizational behavior research. In J. Greenberg (Ed.), Organizational behavior: The state of the science (pp. 327–371). Lawrence Erlbaum Associates. Google Scholar
- Fisher, G. G., Matthews, R. A., & Gibbons, A. M. (2016). Developing and investigating the use of single-item measures in organizational research. Journal of Occupational Health Psychology,21(1), 3–23. ArticlePubMedGoogle Scholar
- Flake, J. K. (2021). Strengthening the foundation of educational psychology by integrating construct validation into open science reform. Educational Psychologist,56(2), 132–141. https://doi.org/10.1080/00461520.2021.1898962ArticleGoogle Scholar
- Flake, J. K., & Fried, E. I. (2020). Measurement schmeasurement: Questionable measurement practices and how to avoid them. Advances in Methods and Practices in Psychological Science,3(4), 456–465. https://doi.org/10.1177/2515245920952393ArticleGoogle Scholar
- Flake, J. K., Pek, J., & Hehman, E. (2017). Construct validation in social and personality research: Current practice and recommendations. Social Psychological and Personality Science,8(4), 370–378. https://doi.org/10.1177/1948550617693063ArticleGoogle Scholar
- Flesch, R. (1948). A new readability yardstick. Journal of Applied Psychology,32(3), 221–233. ArticlePubMedGoogle Scholar
- Fowler Jr., F. J. & Cosenza, C. (2009). Design and evaluation of survey questions. In The SAGE handbook of applied social research methods (pp. 375–412). SAGE Publications, Inc.
- Fowler, F. J. (1995). Improving survey questions. Sage. Google Scholar
- Freedle, R. (2003). Correcting the SAT’s ethnic and social-class bias: A method for reestimating SAT scores. Harvard Educational Review,73(1), 1–44. ArticleGoogle Scholar
- Freedle, R., & Kostin, I. (1992). The prediction of GRE reading comprehension item difficulty for expository prose passages for each of three item types: Main ideas, inferences and explicit statements (GRE Board Professional Report 87–10P; ETS RR-91-59). Educational Testing Service. Google Scholar
- Freedle, R., & Kostin, I. (1997). Predicting Black and White differential item functioning in verbal analogy performance. Intelligence,24(3), 417–444. ArticleGoogle Scholar
- Fuchs, C., & Diamantopoulos, A. (2009). Using single-item measures for construct measurement in management research: Conceptual issues and application guidelines. Die Betriebswirtschaft,69(2), 195–210. Google Scholar
- Furnham, A. (2008). Relationship among four Big Five measures of different length. Psychological Reports,102(1), 312–316. ArticlePubMedGoogle Scholar
- Gehlbach, H., & Brinkworth, M. E. (2011). Measure twice, cut down error: A process for enhancing the validity of survey scales. Review of General Psychology,15(4), 380–387. ArticleGoogle Scholar
- Gibson, A. M., & Bowling, N. A. (2020). The effects of questionnaire length and behavioral consequences on careless responding. European Journal of Psychological Assessment,36(2), 410–420. ArticleGoogle Scholar
- Gilbert, S., & Kelloway, E. K. (2014). Using single items to measure job stressors. International Journal of Workplace Health Management,7(3), 186–199. ArticleGoogle Scholar
- Göritz, A. S (2014). Determinants of the starting rate and the completion rate in online panel studies. In M. Callegaro, R., Baker, J., Bethlehem, A. S., Göritz, J. A., Krosnick, & P. J. Lavrakas (Eds.) Online panel research: A data quality perspective (pp. 154–170). Wiley.
- Guion, R. (1965). Personnel testing. McGraw-Hill. Google Scholar
- Haladyna, T.M., & Rodriguez, M.C. (2013). Developing and validating test items (1st ed.). Routledge.
- Hayes, A. F. (2018). Partial, conditional, and moderated moderated mediation: Quantification, inference, and interpretation. Communication Monographs,85(1), 4–40. ArticleGoogle Scholar
- Heggestad, E. D., Scheaf, D. J., Banks, G. C., Monroe Hausfeld, M., Tonidandel, S., & Williams, E. B. (2019). Scale adaptation in organizational science research: A review and best-practice recommendations. Journal of Management,45(6), 2596–2627. ArticleGoogle Scholar
- Hinkin, T. R. (1998). A brief tutorial on the development of measures for use in survey questionnaires. Organizational Research Methods,1(1), 104–121. ArticleGoogle Scholar
- Hinkin, T. R., & Tracey, J. B. (1999). An analysis of variance approach to content validation. Organizational Research Methods,2(2), 175–186. ArticleGoogle Scholar
- Hu, L. T., & Bentler, P. M. (1999). Cutoff criteria for fit indexes in covariance structure analysis: Conventional criteria versus new alternatives. Structural Equation Modeling: A Multidisciplinary Journal,6(1), 1–55. ArticleGoogle Scholar
- Huang, J. L., Curran, P. G., Keeney, J., Poposki, E. M., & DeShon, R. P. (2012). Detecting and deterring insufficient effort responding to surveys. Journal of Business and Psychology,27(1), 99–114. ArticleGoogle Scholar
- Hughes, D. J. (2018). Psychometric validity: Establishing the accuracy and appropriateness of psychometric measures. In P. Irwing, T. Booth & D. J. Hughes (Eds.), The Wiley handbook of psychometric testing: A multidisciplinary approach to survey, scale and test development. Chichester, UK: Wiley.
- Kincaid, J. P., Fishburne, R. P., Rogers, R. L., & Chissom, B. S. (1975). Derivation of new readability formulas (automated readability index, fog count and Flesch reading ease formula) for Navy enlisted personnel, Research Branch Report 8–75, Millington, TN: Naval Technical Training, U. S. Naval Air Station, Memphis, TN.
- Koo, T. K., & Li, M. Y. (2016). A guideline of selecting and reporting intraclass correlation coefficients for reliability research. Journal of Chiropractic Medicine,15(2), 155–163. ArticlePubMedPubMed CentralGoogle Scholar
- Lapierre, L. M., Matthews, R. A., Eby, L. T., Truxillo, D. M., Johnson, R. E., & Major, D. A. (2018; Focal Article). Recommended practices for initiating and managing research partnerships with organizations. Industrial and Organizational Psychology: Perspectives on Science and Practice, 11(4), 543–581.
- Lian, H., Ferris, D. L., & Brown, D. J. (2012). Does power distance exacerbate or mitigate the effects of abusive supervision? It depends on the outcome. Journal of Applied Psychology,97(1), 107–123. ArticlePubMedGoogle Scholar
- Maul, A. (2017). Rethinking traditional methods of survey validation. Measurement: Interdisciplinary Research and Perspectives, 15(2), 51–69.
- McDonald, R. P. (1999). Test theory: A unified approach. Erlbaum. Google Scholar
- McGonagle, A. K., Huang, J. L., & Walsh, B. M. (2016). Insufficient effort survey responding: An under-appreciated problem in work and organisational health psychology research. Applied Psychology,65(2), 287–321. ArticleGoogle Scholar
- Meade, A. W., & Craig, S. B. (2012). Identifying careless responses in survey data. Psychological Methods,17(3), 437–455. ArticlePubMedGoogle Scholar
- Messick, S. (1995). Validity of psychological assessment: Validation of inferences from persons’ responses and performances as scientific inquiry into score meaning. American Psychologist,50(9), 741–749. ArticleGoogle Scholar
- Muthén, L. K., & Muthén, B. O. (2018). Mplus user’s guide (8th ed.).
- Nagy, M. S. (2002). Using a single-item approach to measure facet job satisfaction. Journal of Occupational and Organizational Psychology,75(1), 77–86. ArticleGoogle Scholar
- Newman, D. A. (2014). Missing data: Five practical guidelines. Organizational Research Methods,17(4), 372–411. ArticleGoogle Scholar
- Nunnally, J. C. (1978). Psychometric theory (2nd ed.). McGraw-Hill. Google Scholar
- Nunnally, J. C., & Bernstein, I. H. (1994). Psychometric theory (3rd ed.). McGraw- Hill. Google Scholar
- Oakland, T., & Lane, H. B. (2004). Language, reading, and readability formulas: Implications for developing and adapting tests. International Journal of Testing,4(3), 239–252. ArticleGoogle Scholar
- Olson, K. (2008). Double-barreled question. In P. Lavrakas (Ed.), Encyclopedia of survey research methods. Sage.
- Ones, D. S., & Viswesvaran, C. (1996). Bandwidth-fidelity dilemma in personality measurement for personnel selection. Journal of Organizational Behavior,17(6), 609–626. ArticleGoogle Scholar
- Peter, J. P. (1979). Reliability: A review of psychometric basics and recent marketing practices. Journal of Marketing Research,16(1), 6–17. ArticleGoogle Scholar
- Podsakoff, P. M., MacKenzie, S. B., Lee, J. Y., & Podsakoff, N. P. (2003). Common method biases in behavioral research: A critical review of the literature and recommended remedies. Journal of Applied Psychology,88(5), 879–903. ArticlePubMedGoogle Scholar
- Raykov, T. (2011). Evaluation of convergent and discriminant validity with multitrait–multimethod correlations. British Journal of Mathematical and Statistical Psychology,64(1), 38–52. ArticlePubMedGoogle Scholar
- Robinson, S. B., & Leonard, K. F. (2018). Designing quality survey questions. Sage. Google Scholar
- Rogelberg, S. G., & Stanton, J. M. (2007). Introduction: Understanding and dealing with organizational survey nonresponse. Organizational Research Methods,10(2), 195–209. ArticleGoogle Scholar
- Rossiter, J. R. (2002). The C-OAR-SE procedure for scale development in marketing. International Journal of Research in Marketing,19(4), 305–335. ArticleGoogle Scholar
- Rossiter, J. R. (2008). Content validity of measures of abstract constructs in management and organizational research. British Journal of Management,19, 380–388. ArticleGoogle Scholar
- Saris, W. E., Revilla, M., Krosnick, J. A., & Shaeffer, E. M. (2010). Comparing questions with agree/disagree response options to questions with item-specific response options. Survey Research Methods,4(1), 61–79. Google Scholar
- Sarstedt, M., & Wilczynski, P. (2009). More for less? A comparison of single-item and multi-item measures. Die Betriebswirtschaft,69(2), 211–227. Google Scholar
- Scarpello, V., & Campbell, J. P. (1983). Job satisfaction: Are all the parts there? Personnel Psychology,36(3), 577–600. ArticleGoogle Scholar
- Scherbaum, C. A., & Goldstein, H. W. (2008). Examining the relationship between race-based differential item functioning and item difficulty. Educational and Psychological Measurement,68(4), 537–553. ArticleGoogle Scholar
- Schmitt, N. (1996). Uses and abuses of coefficient alpha. Psychological Assessment,8(4), 350–353. ArticleGoogle Scholar
- Schriesheim, C. A., Powers, K. J., Scandura, T. A., Gardiner, C. C., & Lankau, M. J. (1993). Improving construct measurement in management research: Comments and a quantitative approach for assessing the theoretical content adequacy of paper-and-pencil survey-type instruments. Journal of Management,19(2), 385–417. ArticleGoogle Scholar
- Singh, J. (2003). A reviewer’s gold. Journal of the Academy of Marketing Science,31(3), 331–336. ArticleGoogle Scholar
- Slaney, K. L., & Garcia, D. A. (2015). Constructing psychological objects: The rhetoric of constructs. Journal of Theoretical and Philosophical Psychology,35(4), 244–259. ArticleGoogle Scholar
- Smith, G. T., McCarthy, D. M., & Anderson, K. G. (2000). On the sins of short-form development. Psychological Assessment,12(1), 102–111. ArticlePubMedGoogle Scholar
- Spector, P. E., Rosen, C. C., Richardson, H. A., Williams, L. J., & Johnson, R. E. (2017). A new perspective on method variance: A measure-centric approach. Journal of Management,45(3), 855–880. ArticleGoogle Scholar
- Spörrle, M., & Bekk, M. (2014). Meta-analytic guidelines for evaluating single-item reliabilities of personality instruments. Assessment,21(3), 272–285. ArticlePubMedPubMed CentralGoogle Scholar
- Stone-Romero, E. F. (1994). Construct validity issues in organizational behavior research. In J. Greenberg (Ed.), Organizational behavior: The state of the science (pp. 155–179). Lawrence Erlbaum Associates. Google Scholar
- Tabachnick, B. G., & Fidell, L. S. (2013). Using multivariate statistics (6th ed.). Pearson. Google Scholar
- Thurstone, L. L. (1947). Multiple factor analysis. University of Chicago Press. Google Scholar
- Tourangeau, R. (2018). The survey response process from a cognitive viewpoint. Quality Assurance in Education,26(2), 169–181. ArticleGoogle Scholar
- Tourangeau, R., Yan, T., & Sun, H. (2020). Who can you count on? Understanding the determinants of reliability. Journal of Survey Statistics and Methodology,8, 903–931. ArticlePubMedGoogle Scholar
- Wanous, J. P., & Hudy, M. J. (2001). Single-item reliability: A replication and extension. Organizational Research Methods,4(4), 361–375. ArticleGoogle Scholar
- Wanous, J. P., & Reichers, A. E. (1996). Estimating the reliability of a single-item measure. Psychological Reports,78(2), 631–634. ArticleGoogle Scholar
- Wanous, J. P., Reichers, A. E., & Hudy, M. J. (1997). Overall job satisfaction: How good are single-item measures? Journal of Applied Psychology,82(2), 247–252. ArticlePubMedGoogle Scholar
- Wayne, J. H., Michel, J., & Matthews, R. A. (2022). Balancing work and family: A theoretical explanation and longitudinal examination of its relation to spillover and role functioning. Journal of Applied Psychology. https://doi.org/10.1037/apl0001007
- Yarkoni, T. (2010). The abbreviation of personality, or how to measure 200 personality scales with 200 items. Journal of Research in Personality,44(2), 180–198. ArticlePubMedPubMed CentralGoogle Scholar
- Ziegler, M., Kemper, C. J., & Kruyen, P. (2014). Short scales-Five misunderstandings and ways to overcome them. Journal of Individual Differences,35(4), 185–189. ArticleGoogle Scholar
Author information
Authors and Affiliations
- University of Alabama, 361 Stadium Drive, Box 870225, Tuscaloosa, AL, 35487, USA Russell A. Matthews & Yeong-Hyun Hong
- Wayne State University, 5057 Woodward Ave., Suite 8402.3, Detroit, MI, 48202, USA Laura Pineault
- Russell A. Matthews